Shamir’s Secret Sharing (SSS) is a well known tool in the cryptography community. It is used in protocols like SLIP39 and FROST. SSS is popular because of its property of information-theoretic security (it is secure even against adversaries who have infinite computational power).
SSS is a powerful cryptographic tool, but it struggles in terms of flexibility. If a share is lost, it is not easy to issue new ones. Adding new shares is possible if enough shares are brought together to recover the original secret, but what if shareholders do not wish to reveal their shares to each other yet? What if they never want to reveal their secret shares, as would be the case with a threshold multisignature protocol such as FROST?
What if the shareholders distrust each other, or the dealer? How can they be sure their shares, or the shares of others, are valid?
In this article, I’ll cover the basics of how Shamir’s Secret Sharing works. I’ll demonstrate how to extend it with verifiable properties so that the dealer and shareholders need not trust one another. Finally I’ll describe a procedure which can be used to trustlessly issue new shares without recovering the full original secret. All the while, shareholders don’t need to trust each other or the dealer, and learn nothing about the new share they issued.
Notation
Just so we’re all on the same page:
Notation | Meaning |
---|---|
The set of integers mod |
|
Sampling |
|
A deterministic concatenation of |
Polynomials
The basis of Shamir’s Secret Sharing lies in the fundamental theorem of algebra, specifically in the mechanics of polynomials, so we should do some review of this domain before we dive into SSS.
Review
- A polynomial expression is any expression of variables* that involves only addition/subtraction, multiplication/division, and positive-integer exponentiation.
- Usually single-variable polynomials denote the variable as
.
- Usually single-variable polynomials denote the variable as
- The degree of a polynomial is the highest power of the variable used in the expression (with a non-zero coefficient).
- The coefficients of a polynomial are the multipliers alongside each power of the variable when it is in standard form.
- The roots of a polynomial expression are the values of
which cause the expression to evaluate to zero. - Sometimes the roots of a polynomial are complex, meaning they involve numbers which make use of the imaginary number
. Don’t worry, we won’t be dealing with complex numbers today!
- Sometimes the roots of a polynomial are complex, meaning they involve numbers which make use of the imaginary number
- A polynomial function is a function which can be computed by simply evaluating a polynomial on an input
.
* Note that today I’ll be writing exclusively about univariate (single-variable) polynomials, where the variable is denoted
For example,
Polynomials aren’t only written in standard form though. They could be written in factored form, such as
By contrast, a function like
Almost everyone will have at least some experience handling polynomials, as most high school math classes deal extensively in linear (degree
Interpolation
The Fundamental Theorem of Algebra states:
Every non-zero, single-variable, degree
polynomial with complex coefficients has exactly complex roots.
In other words: If a polynomial has degree
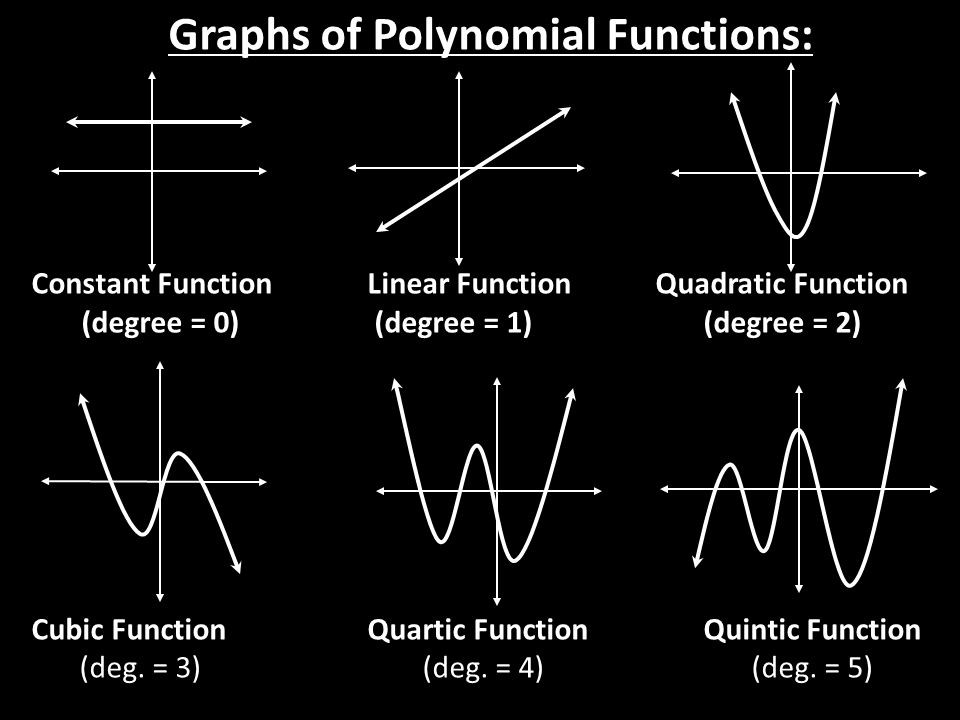
This theorem has a helpful consequence.
Say we have a set of
Uniqueness: Proof by contradiction
How do we know
Assume there exists two non-zero polynomial functions
Since
Consider the difference between the two polynomials
Since
Recall
This is a contradiction. On one hand,
This contradiction is proof that
The very special and helpful property of interpolating polynomials is: To interpolate a polynomial
Said differently, if we create
Perhaps by now you might be starting to see why polynomial interpolation is at the heart of Shamir’s Secret Sharing.
Demonstration
This concept is easier to understand visually with linear polynomials. Picture two points
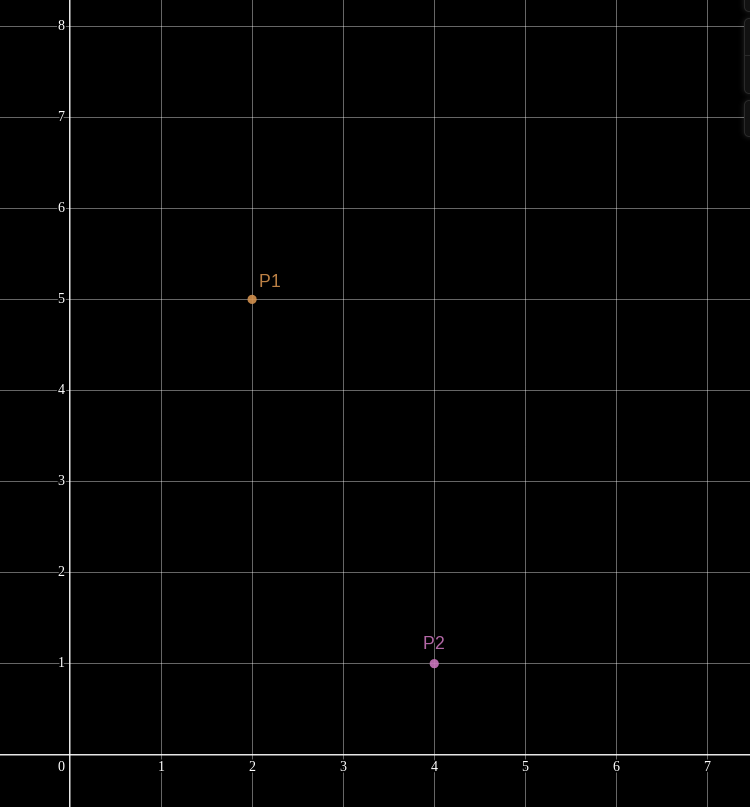
Any two points are sufficient to uniquely describe a line between them. Remember that a line on the Cartesian plane is just a degree-1 (AKA linear) polynomial. Only one unique line (polynomial) passes through (interpolates) the two points.
In this case, the polynomial happens to be
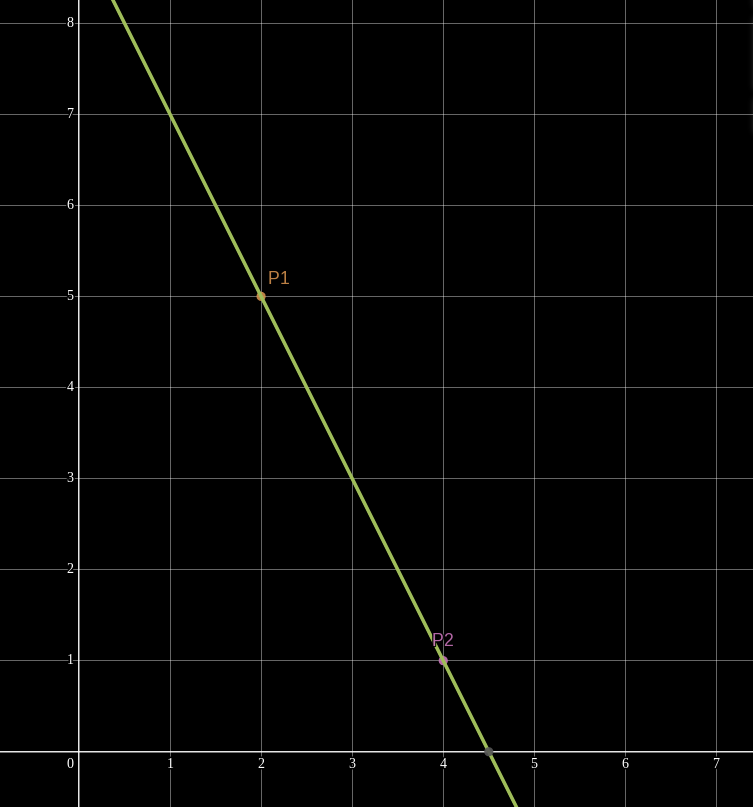
If we are given additional points
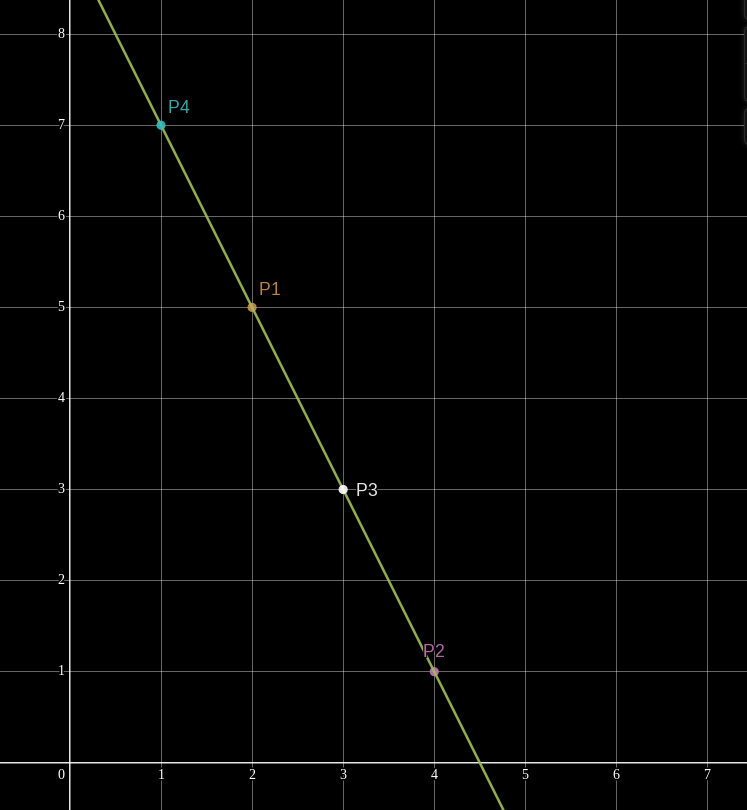
However, if we are given only one evaluation, say
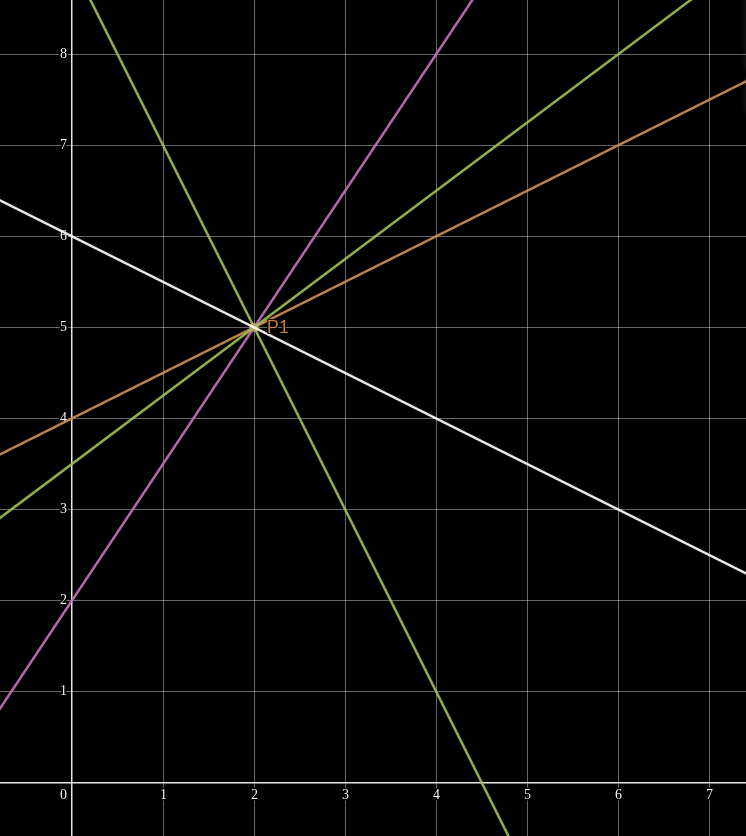
This concept generalizes to higher-degree polynomials! Given the same two points
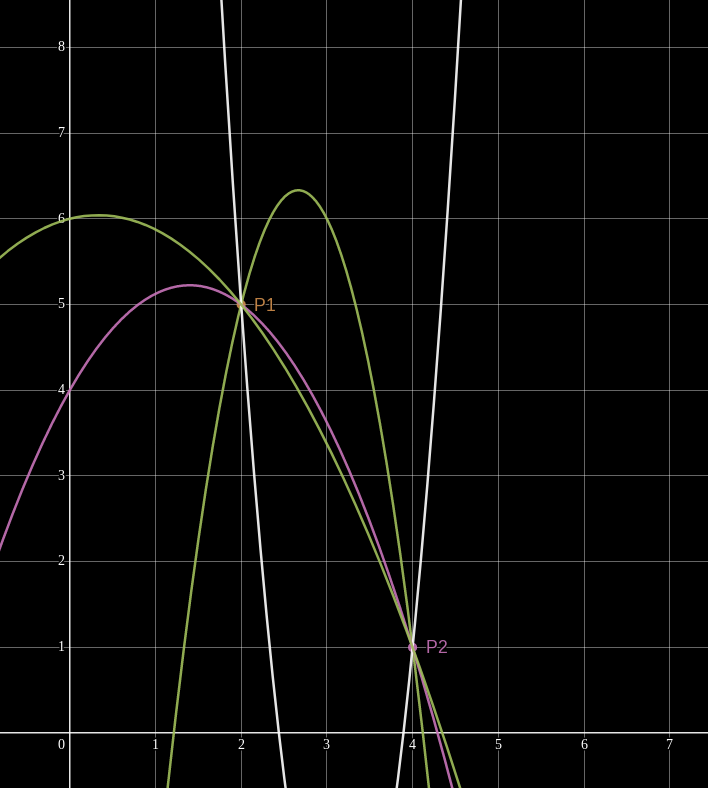
But add a third point and suddenly we can find only one quadratic polynomial which interpolates them.
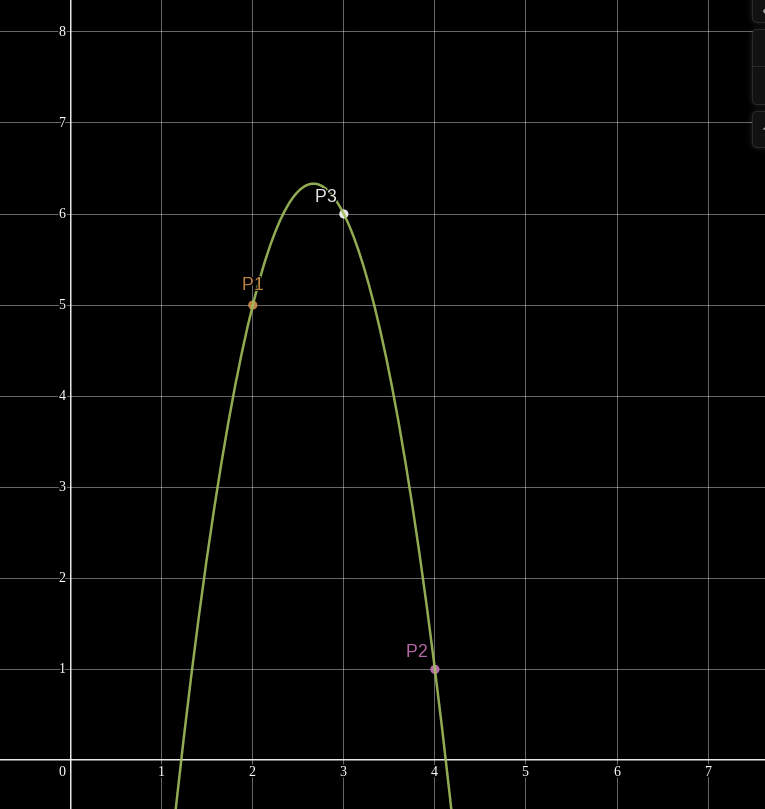
but how would we actually do the interpolation?
There are many ways to skin this cat. Given
- Lagrange Interpolation (the most commonly known)
- Newton Interpolation
- Inverting the Vandermonde Matrix (uses linear algebra)
For the time being, assume we have some black box interpolation algorithm
Shamir’s Scheme
This wouldn’t be a math article if we don’t start by defining some parameters.
- Let
be a Finite Field containing elements, where is some large prime number. - If you’re not familiar with Finite Fields, just consider it as a special group of numbers, such that addition, subtraction, multiplication, and division all work normally as they do within the real numbers, except there are a finite quantity of
possible discrete numbers that can be used. - Usually, this is the set of integers modulo a prime number
, AKA .
- If you’re not familiar with Finite Fields, just consider it as a special group of numbers, such that addition, subtraction, multiplication, and division all work normally as they do within the real numbers, except there are a finite quantity of
- Let
be a secret such that . Our goal is to break into shares so we can distribute them. - Usually
is just some large random number randomly sampled from , such as a private key.
- Usually
- Let
be the total number of shares we want to issue. - Let
be the minimum number of shares required to reconstruct , such that .
Distribution
Shamir’s Secret Sharing scheme starts with a trusted* dealer who knows
* There are other schemes which allow mutually distrustful parties to generate shares of a common but yet-unknown secret, but that is out of scope for today.
The dealer samples
random values from . The dealer treats
as the coefficients of a degree polynomial function .
The dealer assigns each share a unique index
, labeling each share . They could be randomly selected, but usually indexes are just the ascending natural numbers, so we’d have . The indexes must not be zero and must not have repetitions. The dealer evaluates
at each of the different indexes.
- The dealer distributes shares as tuples of
. is the private data within each share which must be kept secret.
Recovery
At recovery time, the original secret
Let
Each of the shares in
* Care is needed here. If the recoverer is malicious, they might use
The recoverer is given a set of
or more shares . Shares must have unique indexes. The recoverer treats the shares as the evaluations produced by some degree
polynomial function over , such that for all . The recoverer uses a polynomial interpolation algorithm
to interpolate the polynomial . The recoverer evaluates
. This outputs , because:
Gotchas
SSS seems simple, but it is an easy protocol to screw up in practice.
- It is very important that the non-constant coefficients of
are totally random, otherwise the information-theoretic security of SSS is lost. - When distributing shares, it is crucial that the dealer does not distribute a share evaluated at index zero, because since
, they would be giving away the secret . - Shareholders must be trusted not to use phony shares when recovering. If one shareholder submits a phony or faulty share, they could change the secret which is recovered to something unusable, and nobody would be able to tell which shareholder screwed up.
- There must be a trusted recoverer machine and a trusted dealer machine who will both know
. If either is compromised, will naturally be exposed. - The dealer and the recoverer must use the same finite field
, which could result in incompatibilities if this field is non-standard.
But still, the power of SSS is undeniable, especially as a primitive when combined with other tools.
Before we demonstrate how shareholders can trustlessly issue new shares, I want to take a moment to learn about how
Interpolation
In my opinion, the most intuitive interpolation method is Lagrange Interpolation. First discovered in 1779 by Edward Waring, this method is named after the scientist Joseph-Louis Lagrange who made the method famous.
The Lagrange method takes a step-by-step approach to achieve a polynomial with all of our desired qualities. Given
- interpolates all
points ( ) - has degree at most
Where to begin? We can make use of the Fundamental Theorem of Algebra once more: A degree
Since we are given
This is the simplest polynomial we could define with roots at
Seems weird to do this, but
Specifically, we can scale
Let’s define this operation as a new polynomial function
As a result,
We can expand
Imagine if we repeat this for all
More commonly, this is written in the mathematical literature in terms of a basis polynomial
Notice how
A common way that modern academic papers will represent the basis polynomials
Example
Here we explore three basis polynomials for the
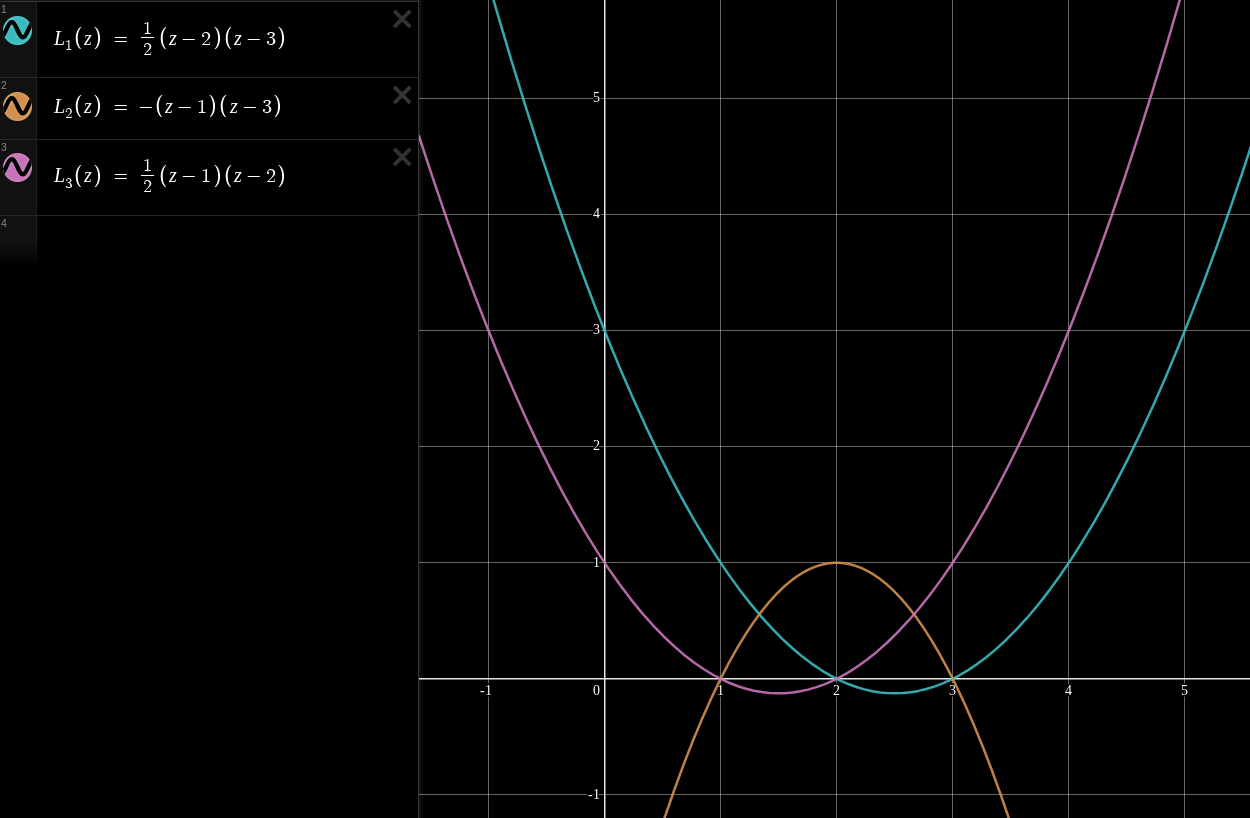
Let’s say we are given the
We can compute our interpolated polynomial on any input
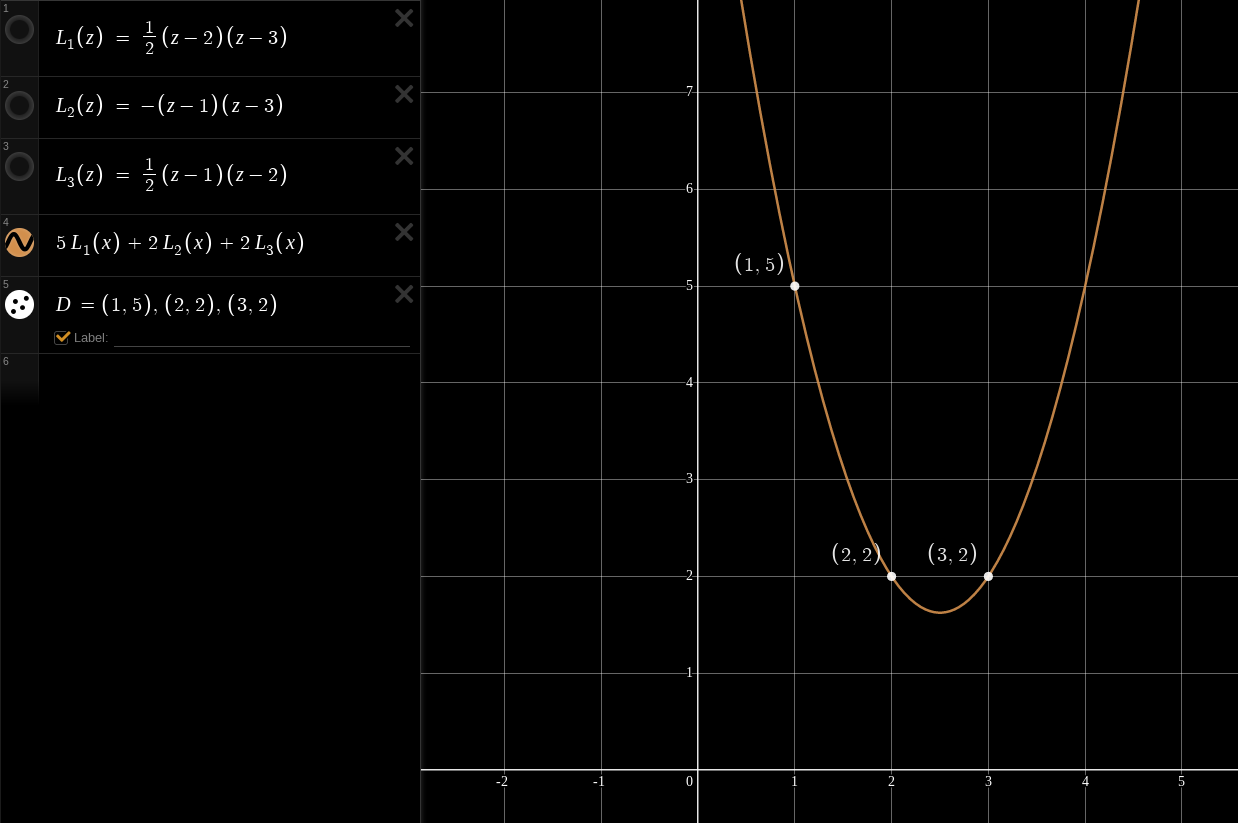
This straightforward method is at the heart of numerous clever algorithms, including Shamir’s Secret Sharing.
There are other improvements we could make to speed this procedure up and increase accuracy for floating point arithmetic - Read about the Barycentric Form of Lagrange Polynomials for more.
But for our purposes this is sufficient background knowledge to proceed to the next section. What I really want you, dear reader, to remember, is how the basis polynomials
- We can give any polynomial a root at any constant
by multiplying a polynomial by . - When we add two polynomials
and together, the roots of either function take the value of its counterpart instead. If , then is the same as .
We will make use of these properties in the next section.
Trustless Sharing
Not so well-known as Shamir’s scheme is Feldman’s Verifiable Secret Sharing (VSS) scheme. This variant of Shamir’s scheme allows participants who distrust each other or the dealer to verify shares are evaluations for a given secret. This turns out to be a very useful property for trustless protocols like multisig.
For Feldman’s VSS, we must introduce a group generator
In principle, this works for any kind of discrete-log group, including multiplicative groups of integers modulo any prime number
Distribution
For Feldman’s VSS extension, the dealing phase of SSS is modified as follows.
- After computing the coefficients
, the dealer computes a set of commitments to the secret and every other coefficient , such that
Notice that
While distributing the shares, the dealer broadcasts
to all shareholders, in such a way that shareholders can be sure they all received the same commitment from the dealer. If no secure broadcasting message system is available, the shareholders must compare with one-another independently to verify they all hold the same values of . Each shareholder can verify their share
is valid under by testing that . This works because act like public equivalents of the secret coefficients which define the dealer’s polynomial .
Knowing
This way, shareholders don’t need to trust the dealer to distribute shares fairly. Perhaps the dealer might be unfair, or faulty. This gives shareholders a way to confirm with each other that they all hold shares of a secret, and that with at least
Recovery
This verification can also be done at recovery time by the recoverer. The recovery process can be modified as follows:
When receiving shares, each share is sent as
where is the original commitment as known to the bearer of share . Each share
is verified against during recovery. All reported commitments must be the same ( holds for any and ).
Any minority of shareholders who submit mismatching commitments or invalid shares can be detected. If all shareholders in
Shares as Keys
If we treat each share
This gives each shareholder a way to prove they own a given share without revealing it: Just sign a message with the secret key
Issuing a New Share
Suppose a dealer has issued
Suppose that a quorum of shareholders possessing at least
- A existing share was lost in a boating accident.
- A existing shareholder was hit by a bus and nobody can find her share.
- A new shareholder is to be inducted into the group.
The naive method would be to bring those
However this comes with a major drawback: There must be a trusted recoverer who reconstructs the full polynomial
Ideally, shareholders should be able to issue new shares without reconstructing
Disclaimer
Don’t implement this in a production environment!
Although I believe I have effectively proven the security of this protocol below, there may be some loophole which would allow a malicious shareholder to learn more about
The Mission
There is a set of
Let
Each bearer of share
Assumptions
For simplicity I may write as though each share at index
It is crucial that each shareholder of
Strategy
In this section I’ll describe the strategy we’ll use to attack this problem at a high level, and hopefully motivate the concept.
Consider a blinding polynomial
Now consider the issue polynomial
Our strategy will be for participants in
Participants in
The question then becomes: How can the issuers in
Multi-Party Computation
All shareholders in
We can use multi-party computation practices to construct a joint polynomial which all shareholders in
Each shareholder
This ensures that the joint blinding polynomial
Procedure
Beware: The procedure I describe in this section is unverifiable for old and new shareholders alike. Shareholders must trust each other not to attempt denial-of-service or griefing attacks by issuing faulty contributions to the multi-party computation. I’ll discuss how to make this fully trustless in the next section.
- Shareholder
samples random coefficients and uses them to construct their partial blinding polynomial as follows.
Shareholder
computes for every other share , and distributes each to the bearer of share . Each bearer of share
waits to receive all - the evaluations of other polynomials on his index . Once in possession of all
, the shareholder can compute by summing all .
- Remember how we defined
? The bearer of share sums and to yield . This is because the share is just the evaluation of , as mentioned earlier.
Each shareholder
sends the evaluation to the new shareholder of index , for a total of points . The new shareholder runs the interpolation algorithm
to yield the degree polynomial , which they can compute at to learn their new share .
At this point, each shareholder
Caution!
Practically,
Verifiable Procedure
The above protocol works in the sense that shareholder
Assume for additional safety that the secret-sharing dealer used VSS, and so the public coefficients
- Once shareholder
has sampled coefficients for their partial blinding polynomial , they compute public commitments for those coefficients .
- Before evaluating any
, shareholder broadcasts their blinding commitment to all other shareholders . In turn, they receive blinding commitments .
Any commitment
A shareholder possessing all
With this, one can compute
It’s important that every
- Once all* other shareholders’ commitments
are in hand and are universally agreed upon, only then does shareholder compute for all and send each evaluation to shareholder .
* For better efficiency, it is okay for shareholder
- Upon receiving the partial blinding polynomial evaluation
from another shareholder , she verifies that the evaluation matches the commitment .
- Shareholders aggregate their partial blinding polynomial evaluations to compute
as described in the previous section.
- Each shareholder
sends to the new inductee shareholder the following data:
- The public coefficients of the share polynomial
: . - The public coefficients of blinding polynomial
: - The new share index
. - The evaluation of the issuance polynomial
.
- The new shareholder
verifies all the things.
- Ensure that the sets of public coefficients
and for the joint polynomials, as well as the new index given by every shareholder in are all consistent. If not, someone might be trying to play funny games. - (optional) If a-priori knowledge of the public point
is available or verifiable, now would be a good time to check that it matches . - Verify each evaluation
is authentic for the given commitments and .
If these checks pass, the new shareholder
- Once the new shareholder has
valid evaluations of , they can use the points to perform polynomial interpolation to recover the full degree polynomial .
Security Proof
I’m now going to take a stab at proving the security of this protocol. Of course, all this assumes the discrete logarithm problem is hard, and
Consider a situation in which a dishonest cabal of malicious shareholders
Shamir’s Secret Sharing is perfectly secure against
Honest Inductee
Consider when
The only new information which
But this is of no use to
Since the new shareholder
Malicious Inductee
Consider an alternative situation in which the new shareholder
First,
Let’s extract the values which are unknown to
Using these evaluations,
Grouping once more to see values known to
In summary,
…with six unknowns:
This is an undetermined system of equations: It has infinite solutions (technically not infinite solutions, since we’re working in the finite field
Trickery
Can
Aside from verification commitments, the only inputs which either honest shareholder accepts from
These potentially malicious inputs are summed with honest partial blinding evaluations from the two honest shareholders to compute the evaluations
Since all shareholders in
Rogue Blinding Evaluations
The blinding polynomial commitments
Under certain conditions, a malicious cabal
Shareholder
If verifiable commitments
This fools shareholder
The new shareholder
Note that for efficiency reasons, it would be entirely safe for shareholder
Security Intuition
Recall we defined
Thanks to
Notice that as long as at least one participant sampled her
Unfortunately for the malicious inductee, every coefficient in
Otherwise, the malicious inductee could have generated the coefficients of
Degrees of Freedom
It is important that the blinding polynomial
If
On the other hand,
If the new shareholder
Blinding Polynomial Secrecy
It is crucial that the full blinding polynomial
If
The joint blinding polynomial coefficients
Here's how.
If two distinct issuance polynomials
Let
For brevity, let’s define
Phrase
This will work out similarly for the alternate issuance polynomial.
Set both sides equal.
Together the colluding shareholders know
Index Verification
The shareholders in
It is also important that each shareholder can positively identify which agents holds which shares when communicating. Agents who misrepresent their share indexes could fool honest shareholders into computing erroneous data.
For example, if a potential new shareholder asks to be issued a share at index
Shareholders who hold multiple shares may equivocate by reporting different share indexes to different peers during the setup phase. If shareholder
Use Cases
Recovery
Share recovery is probably the most realistic use case for this algorithm. If shareholders maintain a record of who has which share, then they can safely recover lost shares. Records of share ownership are crucial here, so that a phony recovery attempt does not give a malicious shareholder access to new shares they shouldn’t have. For example, Bob might have share
As an example, say an employee’s laptop is wiped with a Shamir share share on it. The other staff on their team who - assuming they have at least
Users of SLIP39 might find this procedure interesting, as it would allow a user to recover lost SLIP39 shares without having to physically bring the shares together. Yet, I doubt the security of this concept in practice, given the highly sensitive nature of a Bitcoin hardware wallet. To perform interactive share recovery with multi-party computations, the threshold of shares would need to be plugged into separate internet-connected computers, which defeats the purpose of a hardware wallet in the first place.
Interactive share recovery (and all the verification steps) would need to be implemented in hardware wallet firmware, and then all
Repairable Multisignature
In the case of multisignature, the share recovery protocol would allow signers in polynomial-based threshold signing systems like FROST to recover the long-lived signing shares of signers who experienced some hardware fault or forgot the encryption password for their signing key.
They could also enroll new signers into their group, provided they can mitigate the risk that a new signer might actually be an old signer in a fake mustache.
Repairable Escrow
One could create a redundant 2-of-3 multisignature escrow service in which the user acts as the dealer of shares of a threshold private key. One share (the escrow share) is given to the escrow agent, and two are given to the user. The user can store one of their shares (the cold share) on paper or a USB drive, somewhere safe and offline. The other share (the hot share) can be an online, active-use signing key, used to collaborate with the escrow agent to sign certificates, Bitcoin transactions, emails, etc.
- The escrow agent acts as a remote second factor, verifying the user with some secondary authentication method like a hardware token, or biometrics.
- If the user loses one of their shares but retains the other share, the escrow agent can collaborate with the user to recover the lost share trustlessly, thereby returning the user to his original fully functioning state. Other threshold multisignature schemes would require a key migration to fully recover both of the user’s shares.
- If the escrow agent disappears, the user can independently recover their share with the hot and cold shares, and give the recovered escrow share to a new escrow agent. Colluding escrow agents would be powerless, because they each have the same share.
Alternative Approaches
Sampling Evaluations
One could also design the partial blinding polynomials
A shareholder
They would append the fixed point
The evaluations
This alternative approach is a helpful demonstration that however
NSG Enrollment
This paper by Stinson and Wei describes a similar approach to issue or recover Shamir shares, in section 2. Unfortunately I only read about this method once I had already finished the majority of this article.
Both methods are very similar and share similar security proofs. Stinson and Wei’s approach might be slightly more efficient in terms of computations, but they also did not describe a verification process to avoid denial-of-service and griefing attacks.
Conclusion
Other interesting extensions to Shamir’s Secret Sharing include changing the threshold, removing a shareholder, or modifying the shared secret in a verifiable fashion.
I might spend a bit of time to write a demo implementation of this algorithm in Python or Rust in the near future if there is sufficient interest.
I have seen some recent interest on the concept of hardware-backed FROST signing tools. Having the ability to recover such a device without the need to migrate keys would be a huge win.
Sources
- A Practical Scheme for Non-Interactive Verifiable Secret Sharing - Paul Feldman
- SLIP39 Specification - SatoshiLabs
- FROST: Flexible Round-Optimized Schnorr Threshold Signatures - Komlo & Goldberg
- Combinatorial Repairability for Threshold Schemes - Stinson & Wei
- Fundamental Theorem of Algebra - Wikipedia
- Lagrange Polynomials - Wikipedia